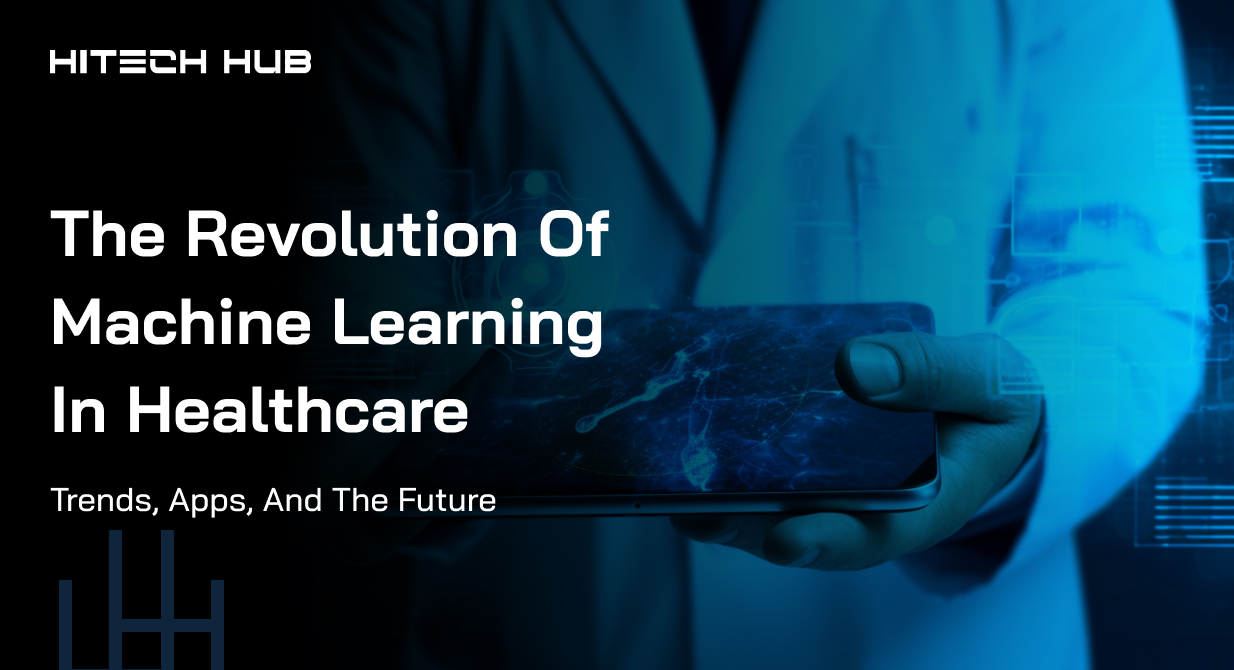
The Revolution of Machine Learning in Healthcare: Trends, Apps, & the Future
Machine learning (ML) has emerged as a transformative force in healthcare, driving innovations that improve patient outcomes, streamline operations, and enhance the overall efficiency of the industry. As technology continues to advance, the integration of machine learning into healthcare practices is reshaping how we approach medical diagnostics, treatment plans, and patient care. In this blog post, we’ll explore the latest trends in machine learning for healthcare, highlight the top health tech apps leveraging these advancements, and discuss how these developments are setting the stage for the future of medicine.
What Statistics You Should Know About Healthcare:
- In 2019, the global digital health market was worth an estimated 175 billion U.S. dollars. With an expected CAGR of almost 25 percent from 2019 to 2025, the digital health market should reach nearly 660 billion dollars by 2025.
- In 2021, eight of the largest ten digital health investment deals were done in the United States. In this year, 600 million U.S. dollars were invested into the U.S. company Ultima Genomics making it the largest deal. The largest investment involving a company from outside the U.S. was concerning Doctolib which received a deal of 549 million U.S. dollars.
Recent Trends in Machine Learning for Healthcare
1. Predictive Analytics for Personalized Medicine
One of the most significant trends in healthcare is the use of predictive analytics powered by machine learning to personalize treatment plans. Predictive models analyze vast amounts of data from electronic health records (EHRs), genetic information, and lifestyle factors to forecast an individual’s risk of developing certain conditions. For instance, ML algorithms can predict the likelihood of a patient developing chronic diseases such as diabetes or cardiovascular conditions, allowing for early intervention and personalized prevention strategies.
2. Advanced Diagnostic Tools
Machine learning is increasingly being used to develop advanced diagnostic tools that improve accuracy and speed in identifying diseases. Techniques such as deep learning and image recognition are particularly impactful in radiology, where ML models can analyze medical images (e.g., X-rays, MRIs, CT scans) to detect abnormalities with remarkable precision. For example, ML algorithms are now capable of identifying early signs of cancer, often before they become visible to the human eye, leading to earlier and more effective treatment.
3. Natural Language Processing (NLP) in Healthcare
Natural Language Processing (NLP) is another crucial area where machine learning is making strides. NLP enables computers to understand, interpret, and generate human language, which is particularly useful for processing unstructured data in clinical notes, research papers, and patient interactions. By leveraging NLP, healthcare providers can extract valuable insights from vast amounts of textual data, improving decision-making processes and streamlining administrative tasks.
4. Robotic Process Automation (RPA) for Administrative Efficiency
Robotic Process Automation (RPA) combined with machine learning is being utilized to enhance administrative efficiency in healthcare settings. RPA systems automate repetitive tasks such as appointment scheduling, claims processing, and patient data entry, reducing the administrative burden on healthcare professionals. This automation not only minimizes human error but also allows healthcare staff to focus more on patient care.
5. Telemedicine and Remote Monitoring
The rise of telemedicine and remote monitoring solutions has been accelerated by machine learning technologies. ML algorithms are used to analyze data from wearable devices and remote sensors, providing real-time insights into a patient’s health status. This capability is particularly beneficial for managing chronic conditions and facilitating ongoing patient monitoring without the need for frequent in-person visits.
Top 5 Healthtech Apps Revolutionizing Healthcare with Machine Learning
1. IBM Watson Health
IBM Watson Health is a leading example of how machine learning is revolutionizing healthcare. The app leverages IBM’s powerful AI to assist healthcare providers with data-driven insights and decision support. Watson Health’s capabilities include analyzing medical literature, patient records, and clinical data to offer personalized treatment recommendations and identify potential research opportunities. Its AI-driven approach helps streamline diagnostic processes and improve patient outcomes.
Features:
- AI-powered data analysis
- Personalized treatment recommendations
- Advanced research insights
2. Ada Health
Ada Health is an AI-powered symptom checker that uses machine learning algorithms to analyze user symptoms and provide preliminary diagnostic suggestions. The app leverages a vast database of medical knowledge to offer users insights into potential conditions and recommend appropriate next steps. Ada Health’s approach helps users better understand their health concerns and seek timely medical attention.
Features:
- AI-based symptom checking
- Personalized health insights
- Recommendations for next steps
3. K Health
K Health is another innovative healthtech app that uses machine learning to offer personalized medical advice. By analyzing user symptoms and comparing them with a large dataset of health information, K Health provides users with potential diagnoses and treatment options. The app also connects users with licensed doctors for further consultation, blending AI-driven insights with human expertise.
Features:
- AI-based diagnostic tool
- Personalized treatment options
- Access to licensed doctors
4. PathAI
PathAI focuses on leveraging machine learning to enhance pathology and diagnostic accuracy. The app uses advanced image recognition algorithms to analyze pathology slides, helping pathologists detect diseases such as cancer with greater precision. PathAI’s technology aims to reduce diagnostic errors and improve the overall quality of pathology services.
Features:
- Advanced image recognition
- Enhanced diagnostic accuracy
- Pathology slide analysis
5. MySugr
MySugr is a diabetes management app that utilizes machine learning to help users track their blood sugar levels and manage their diabetes more effectively. The app offers personalized insights based on user data, including blood glucose readings, diet, and activity levels. MySugr’s features include automated logbooks and personalized feedback, which help users stay on top of their diabetes management.
Features:
- Blood sugar tracking
- Personalized diabetes management
- Automated logbooks and feedback
The Future of Machine Learning in Healthcare
As machine learning continues to evolve, its potential applications in healthcare are vast and promising. The future of ML in healthcare will likely involve:
- Integration with Genomic Data: The combination of ML with genomic data will enable even more personalized medicine approaches, tailoring treatments to an individual’s genetic makeup.
- Enhanced Predictive Models: Future predictive models will become increasingly accurate, helping to preemptively address health issues before they manifest.
- Expanded Use of Wearable Technology: Wearables and IoT devices will provide a continuous stream of data, further enhancing remote monitoring and personalized care.
- Improved Natural Language Understanding: Advances in NLP will allow for better interpretation of complex medical texts and patient communications, improving overall healthcare delivery.
Machine learning (ML) offers a range of transformative benefits in healthcare, contributing to improved patient outcomes, operational efficiencies, and overall advancements in medical practice. Here are five key benefits of machine learning in healthcare:
Ready to innovate in healthcare? Contact hi-tech hub and build your app now!
5 benefits of machine learning in healthcare
1. Enhanced Diagnostic Accuracy
Machine learning algorithms can analyze complex medical data with high precision, often surpassing the capabilities of traditional diagnostic methods. By learning from large datasets, ML models can identify patterns and anomalies that may be missed by human observers.
In radiology, deep learning algorithms are used to analyze medical images, such as X-rays and MRIs, for detecting conditions like tumors or fractures. For instance, Google Health’s deep learning model for eye disease diagnosis has demonstrated high accuracy in detecting diabetic retinopathy and age-related macular degeneration (AMD) from retinal scans.
2. Personalized Treatment Plans
Machine learning enables the development of personalized treatment plans by analyzing a patient’s unique medical history, genetic information, and lifestyle factors. This personalized approach helps in tailoring treatments to individual needs, improving effectiveness and reducing adverse effects.
IBM Watson for Oncology uses machine learning to analyze patient data and recommend personalized treatment options based on the latest research and clinical guidelines. This approach helps oncologists design treatment plans tailored to the specific characteristics of each patient’s cancer.
3. Predictive Analytics for Early Intervention
ML models can analyze historical and real-time data to predict the likelihood of developing certain conditions, enabling early intervention and preventive care. Predictive analytics help in identifying at-risk patients before symptoms manifest, allowing for proactive management.
Tools like the Framingham Risk Score, enhanced by ML techniques, predict cardiovascular disease risk based on patient data. By analyzing factors such as age, blood pressure, and cholesterol levels, these models help in early identification and intervention to prevent heart attacks and strokes.
4. Operational Efficiency and Cost Reduction
Machine learning automates routine administrative tasks, optimizes resource allocation, and streamlines workflow processes in healthcare settings. This automation reduces administrative burdens on healthcare professionals and minimizes operational costs.
Robotic Process Automation (RPA) combined with ML is used in tasks such as appointment scheduling, claims processing, and patient data entry. By automating these tasks, healthcare systems can improve efficiency and reduce operational costs, allowing staff to focus more on patient care.
5. Improved Patient Monitoring and Management
Machine learning enhances patient monitoring by analyzing data from wearable devices and remote sensors. This continuous monitoring provides real-time insights into patient health, supporting better management of chronic conditions and timely intervention when needed.
Apps like MySugr use machine learning to track blood glucose levels and provide personalized feedback for diabetes management. The app analyzes data from glucose monitors, dietary inputs, and physical activity to help patients manage their condition more effectively.
Implications for Mobile App Development In Healthcare
As mobile app developers, understanding the impact of machine learning in healthcare can guide the creation of innovative solutions that address real-world needs. Here’s how these insights can be applied in app development:
- Incorporate Advanced Analytics: Leverage ML algorithms to build apps that offer predictive analytics, personalized treatment plans, and real-time health monitoring. Integrate features that analyze user data and provide actionable insights to enhance user engagement and health outcomes.
- Focus on User Experience: Design intuitive and user-friendly interfaces that make complex ML-powered features accessible to users. Ensure that the app’s functionality aligns with user needs and preferences, enhancing overall satisfaction and usability.
- Prioritize Data Security: Implement robust security measures to protect sensitive health data. Compliance with regulations such as HIPAA (Health Insurance Portability and Accountability Act) and GDPR (General Data Protection Regulation) is crucial for maintaining user trust and ensuring data privacy.
- Collaborate with Healthcare Professionals: Engage with healthcare providers and domain experts to validate app functionalities and ensure that ML models are aligned with clinical needs. Collaboration can also facilitate the integration of apps into existing healthcare systems.
- Focus on Continuous Improvement: Machine learning models require ongoing training and refinement. Develop apps with mechanisms for updating algorithms and incorporating user feedback to continuously improve performance and accuracy.
Estimated costs for developing different types of healthcare apps:
App Type | Features | Estimated Cost (USD) |
Basic | – Simple UI/UX – Basic health tracking (e.g., step counting) – Basic user authentication – Basic data storage and retrieval |
$10,000 – $30,000 |
Advanced | – Advanced UI/UX – Integration with wearable devices – Advanced health monitoring (e.g., vitals tracking) – Secure data handling and encryption – API integrations (e.g., with health databases) |
$30,000 – $75,000 |
Complex | – Highly customizable UI/UX – Integration with multiple health devices and systems – Advanced health analytics and reporting – Telemedicine features (e.g., video calls) – Compliance with health regulations (e.g., HIPAA) – Advanced security measures |
$75,000 – $150,000+ |
Bring Your Health App Vision to Life with Hi-tech Hub!
Transform the way patients interact with their health using cutting-edge technology. At Hi-tech Hub, our expert developers are here to turn your vision into a reality. Don’t wait—contact us today to start developing your innovative health app and lead the way in digital health solutions. Reach out now and let’s create something extraordinary together!
Eshika Jain
11-September-2024